VOCALISE
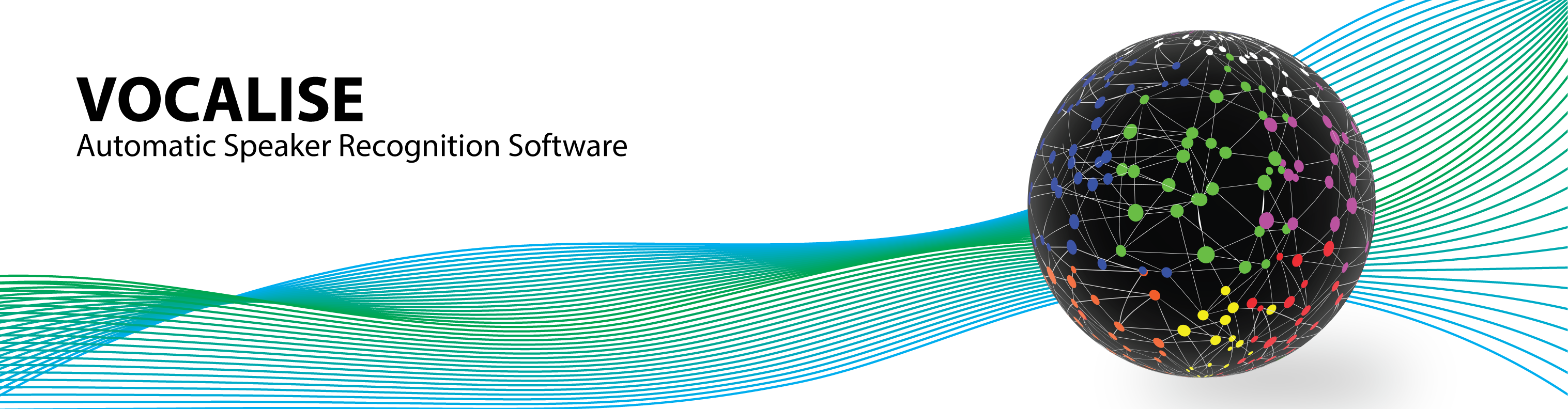
VOCALISE (Voice Comparison and Analysis of the Likelihood of Speech Evidence) is a powerful and flexible voice biometrics software solution for performing fast and accurate speaker comparisons using Deep Neural Network (DNN) based x-vectors.
Multi-speaker file comparison
State-of-the-art x‑vector PLDA
Condition adaptation
Powerful visualisation feature
‘Open-box’ architecture
Score calibration to interpret scores across different conditions
Objective audio quality measures and ‘star rating’
Use objective quality metrics to create subsets of files for analysis
Provided with powerful performance assessment tool
Highlights
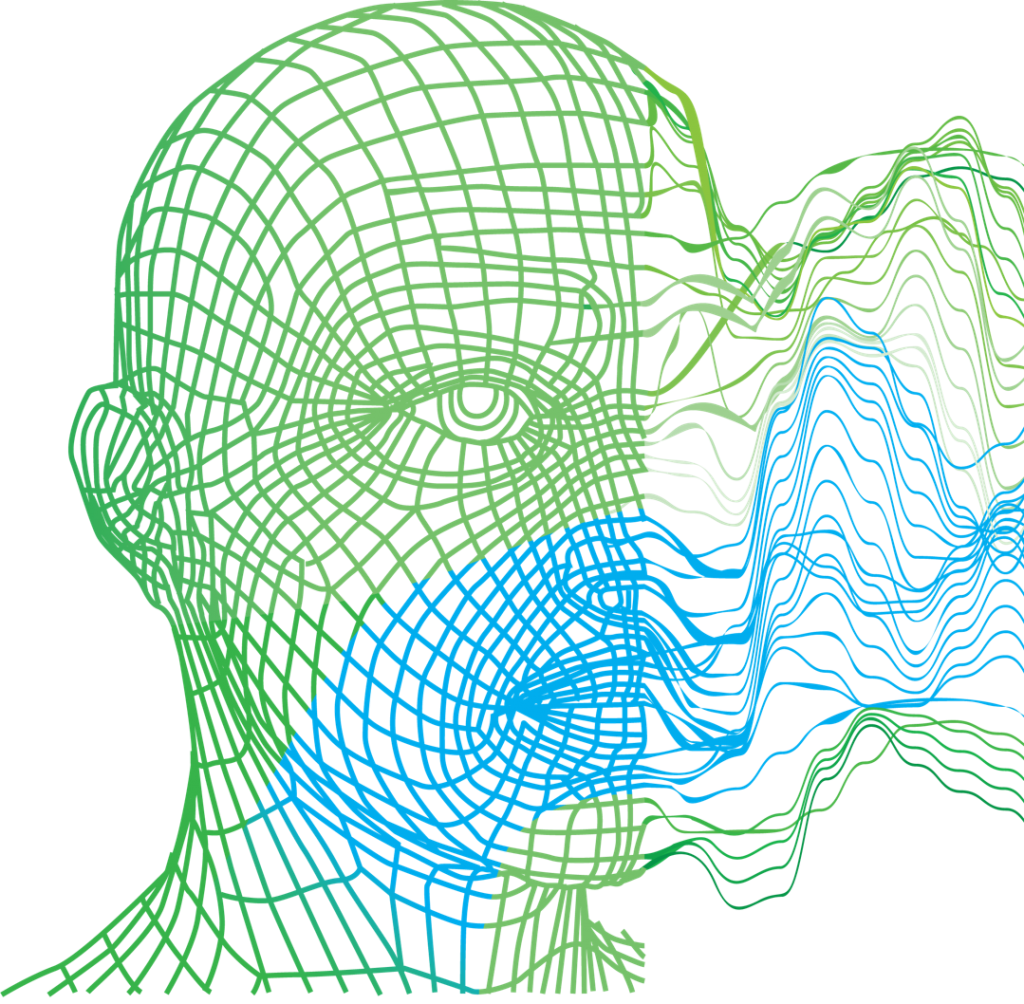
Comparison of multi-speaker audio files without diarisation using a segmental approach, or by easy selection of speech from a speaker of interest
Operates using a state-of-the-art x‑vector PLDA (Probabilistic Linear Discriminant Analysis) framework
Powerful visualisation feature for i‑vector and x‑vector approaches: view an interactive 3D point cloud of speakers
Condition adaptation for ‘tuning’ the i‑vector or x‑vector PLDA system to a particular or unseen recording condition
Supplied with Bio-Metrics software allowing performance assessment via equal error rates, Tippett plots, DET curves, and Zoo plot analysis, and calculation of likelihood ratios under same-speaker and different-speaker hypotheses
Multi-speaker comparisons
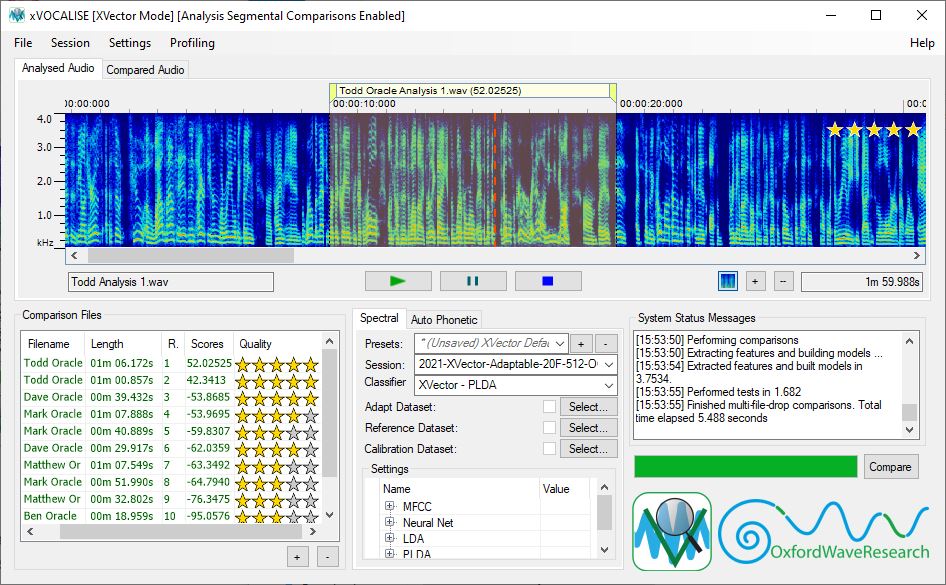
Handle multiple speakers in two ways – VOCALISE now allows you to compare files with more than one speaker in it using these two powerful modes:
Selections
VOCALISE selections allow you to highlight speech of interest and use only that to compare against other files.
Use case: manually select a speaker of interest within a multi-speaker file and send to VOCALISE for comparison.
Segmental mode
The VOCALISE segmental mode allows you to automatically compare single-speaker recordings with multi-speaker recordings, without having to diarise the multi-speaker recording (i.e. separate out the speech of the speaker of interest), and rapidly highlights the regions in the multi-speaker file most likely to contain the speaker of interest.
Audio Management Panel 
- Apply channel conversions and resampling of files for use in VOCALISE, avoiding the need for pre-processing in other software
- Import from some common media formats to VOCALISE
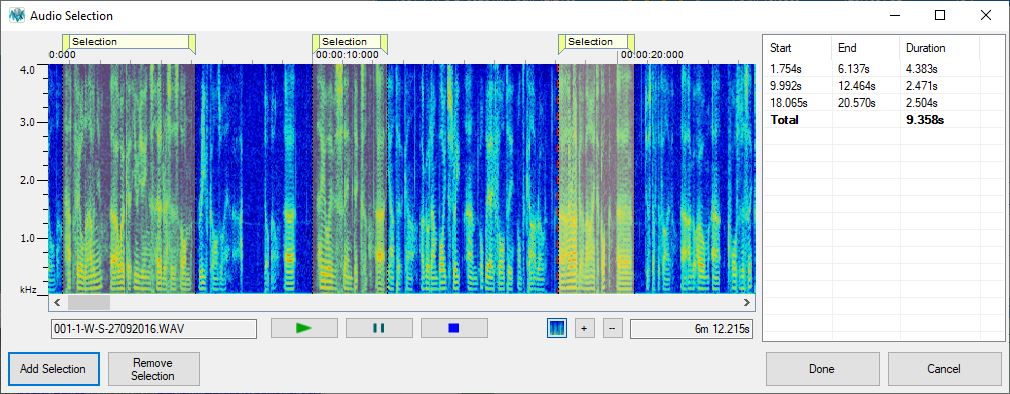
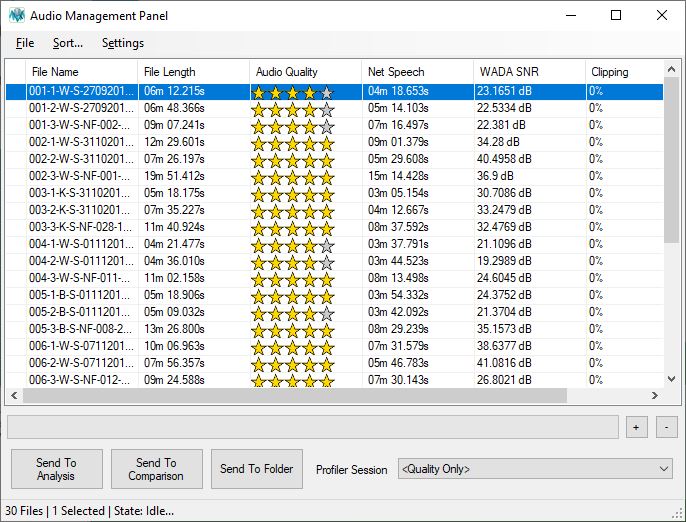
- Obtain an objective quality star rating for audio files that incorporates SNR (signal-to-noise ratio), net-speech duration, and clipping measures.
- Use case: easily select high-quality files for comparison in VOCALISE
State-of-the-art algorithms
VOCALISE supports several generations of automatic speaker recognition technology, from classic approaches based on Gaussian Mixture Models (GMMs), to state-of-the-art i-vectors and x-vectors. Over the course of this technology evolution, the complexity of the systems has increased, as has the recognition performance.
Deep Neural Networks (DNNs) mark a new phase in the evolution of automatic speaker recognition technology, providing a powerful framework for extracting highly-discriminative speaker-specific features from a recording of speech. VOCALISE continues to support both legacy and state-of-the-art speaker modelling algorithms, the latest of which is a DNN-based ‘x-vector’ framework, a state-of-the-art approach that leverages a DNN to extract compact speaker representations.
‘Open-box’ architecture
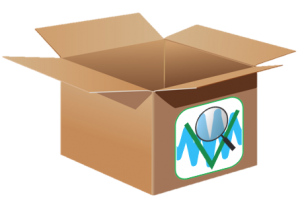
- Ability to view and change the system parameters, and to introduce new data at every step of the speaker recognition process.
- Not limited to manufacturer-provided models or configurations
- Ability to train the system specifically for the user’s problem domain
- Interfacing with ‘trusted’ programs like Praat to utilise features provided by such specialist software
Flexible speaker feature extraction options
Spectral features
Uses Mel-frequency cepstral coefficient (MFCC) features with cepstral mean subtraction (CMS) and variance normalisation (CMVN)
Auto-phonetic features
The use of ‘auto-phonetic’ features, i.e. phonetic features extracted in an automatic (unsupervised) way, is supported via an interface with Praat.
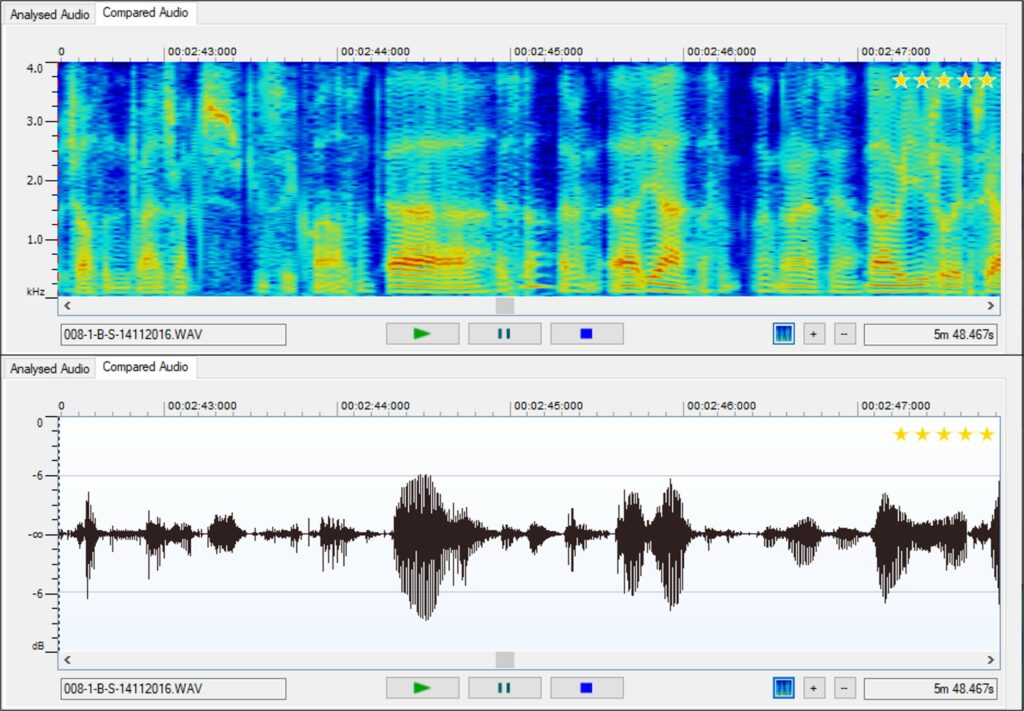
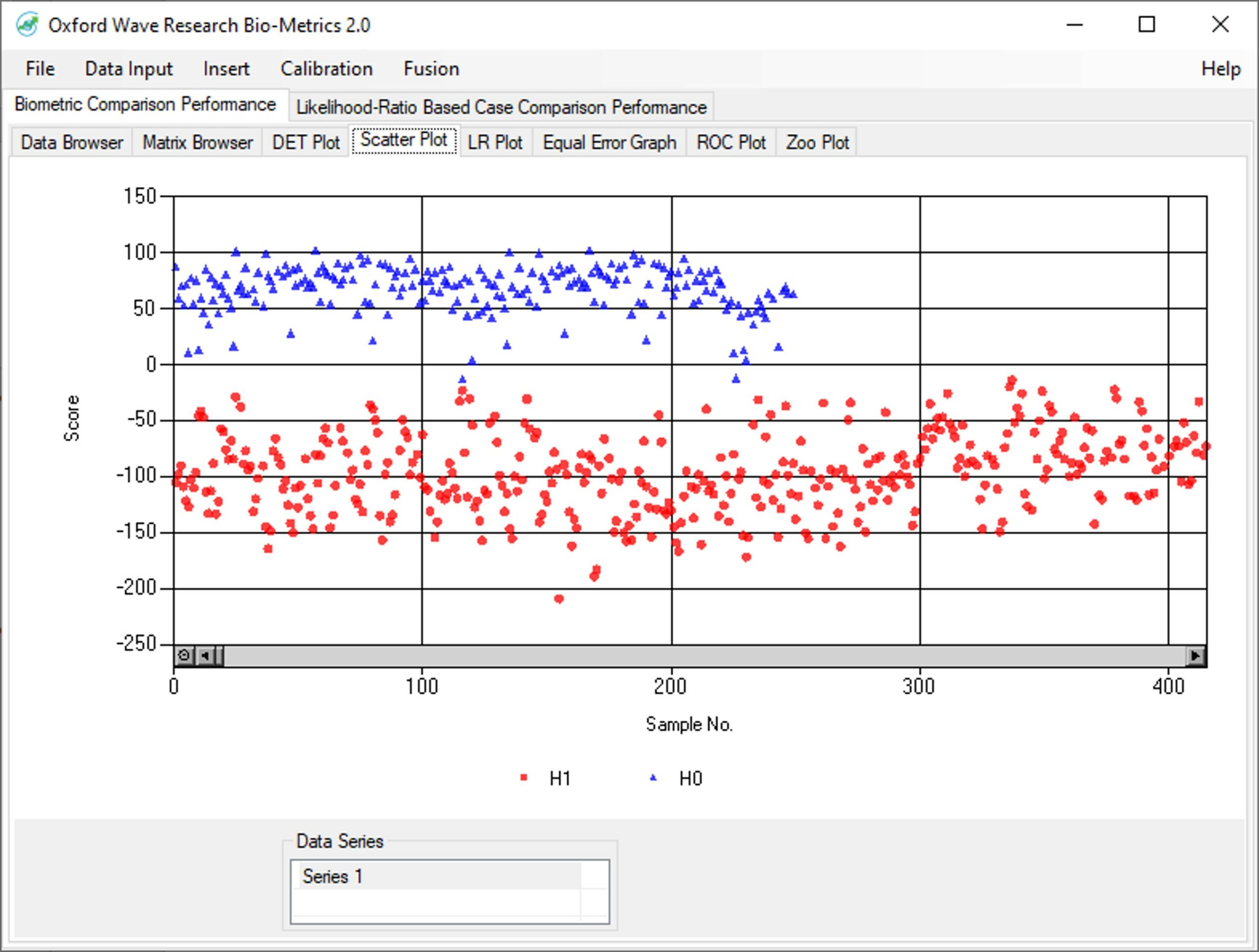
Other features
- Audio playback and visualisation
- Optimised to run quickly on multi-processor machines
- Ready-to-use ‘sessions’ (pre-trained speaker recognition models)
- Easy switching between the auto-phonetic and spectral analysis modes
- Auto-phonetic mode provides the unique capability of running automatic comparisons based on features traditionally used in forensic phonetic acoustic analysis
- Optional Voice Activity Detection (VAD) pre-processing
Use our accompanying Bio-Metrics Software to analyse VOCALISE comparison scores and calculate the strength of evidence based on likelihood ratios for your case in a graphical and interactive way.
Recent publications using VOCALISE
VOCALISE has a large body of scientific literature evaluating and supporting its use both by our users across the world and our own research team. Browse through some of the latest publications and conference presentations below or visit our Publications page for more.
- Linda Gerlach, Kirsty McDougall, Finnian Kelly, and Anil Alexander. Voice twins: discovering extremely similar-sounding, unrelated speakers, Proceedings of INTERSPEECH 2023, Dublin, Ireland, URL. [Download Paper]
- Linda Gerlach, Kirsty McDougall, Finnian Kelly, and Anil Alexander. Automatic assessment of voice similarity within and across speaker groups with different accents, Proceedings of the International Congress of Phonetic Sciences (ICPhS) 2023, Prague, Czech Republic, URL. [Download Paper]
- Vincent Hughes, Jessica Wormald, Paul Foulkes, Philip Harrison, Poppy Welch, Chenzi Xu, Finnian Kelly, and David van der Vloed. Effects of vocal variation on the output of an automatic speaker recognition system, International Association for Forensic Phonetics and Acoustics (IAFPA) conference 2023, pp. 35-36, Zurich, Switzerland. [Download Abstract]
- Chenzi Xu, Paul Foulkes, Philip Harrison, Vincent Hughes, Poppy Welch, Jessica Wormald, Finnian Kelly, and David van der Vloed. Impact of mismatches in long-term acoustic features upon different-speaker ASR scores, International Association for Forensic Phonetics and Acoustics (IAFPA) conference 2023, pp. 33-34, Zurich, Switzerland. [Download Abstract]
- David van der Vloed and Tina Cambier-Langeveld. How we use automatic speaker comparison in forensic practice, International Journal of Speech, Language and the Law, 2023, 29(2), pp. 201-224. https://doi.org/10.1558/ijssl.23955
- Linda Gerlach, Kirsty McDougall, Finnian Kelly, and Anil Alexander. Seeking voice twins – an exploration of VoxCeleb using automatic speaker recognition and two clustering methods, International Association for Forensic Phonetics and Acoustics (IAFPA) conference 2022, pp. 45-46, Prague, Czech Republic. [Download Abstract]
- Finnian Kelly, Harry Swanson, Kirsty McDougall, and Anil Alexander. Classifying non-speech vocalisations for speaker recognition, International Association for Forensic Phonetics and Acoustics (IAFPA) conference 2022, pp. 7-8, Prague, Czech Republic. [Download Abstract]
- Anil Alexander, Finnian Kelly, and Erica Gold. A WYRED connection: x-vectors and forensic speech data, International Association for Forensic Phonetics and Acoustics (IAFPA) conference 2021, Marburg, Germany. [Download Abstract]
- Linda Gerlach, Kirsty McDougall, Finnian Kelly, and Anil Alexander. How do automatic speaker recognition systems ‘perceive’ voice similarity? Further exploration of the relationship between human and machine voice similarity ratings, International Association for Forensic Phonetics and Acoustics (IAFPA) conference 2021, Marburg, Germany. [Download Abstract]
- Linda Gerlach, Tom Coy, Finnian Kelly, Kirsty McDougall, and Anil Alexander. How does the perceptual similarity of the relevant population to a questioned speaker affect likelihood ratio?, International Association for Forensic Phonetics and Acoustics (IAFPA) conference 2021, Marburg, Germany. [Download Abstract]
Contact Us